Today, every company must look to data & analytics to improve decision making for improved business performance. These business decisions could be as simple as giving a discount to a prospect or as complex as structuring a large acquisition. Organizations spend a lot of time and effort managing and analyzing data to draw meaningful conclusions. While this is no small feat, the more challenging task is translating these insights into the right decisions – commonly known as the “last mile” analytics problem.
In today’s environment of uncertainty, making the right decisions is both challenging and critical to remaining competitive. The lines between strategic, tactical, and operational decisions are blurring. Most enterprises are, therefore, plagued with decision paralysis due to unreliable data, poor leadership, and other factors, resulting in missed opportunities and unnecessary costs. Research by Mckinsey found high levels of frustration with inefficient decision-making processes in most companies. Gartner says ineffective and delayed decisions cost firms more than 3% of profits, which is a whopping $270 billion in the U.S. alone.
Most enterprises are plagued with decision paralysis due to unreliable data and poor data & analytics leadership.
So how can business leaders make better decisions? The DEAR model, an acronym for define, establish, analyze, and realize, is a data-driven methodology for improving corporate decision making. I developed this method from extensive research on decision theory, consultation with experts, and experience from a plethora of client engagements around this subject.
This systematic approach is applicable in any situation where leaders must effectively manage the decision-making process. While unstructured decision making is vulnerable to poor decisions, adding structure results in faster, more rational decisions across the organization.
Phase 1: Define
The define phase is about crafting the decision problem. A typical decision problem has four key elements: an objective, alternatives, pay-off, and outcomes.
- Objectives are measurable goals that result in improved performance. There are two primary types of objectives: maximizing and minimizing key variables such as revenue or profit.
- Alternatives are choices between two or more courses of action. While there could be many competing alternatives, the viable or realistic alternatives are those that are under the control of the decision maker and have resources available to realize.
- The payoff is the benefit that is gained from the decision-making process and is often a combination of both tangible and intangible benefits. Basically, the payoff is the gain or loss expected from each outcome.
- The outcome is the end state that results when the decision is implemented.
Phase 2: Establish
The establish phase helps to quantify the payoffs associated with each alternative using quality data and insights. The payoffs are often a combination of both tangible and intangible benefits. Tangible benefits, called “hard savings”, can be quantified financially while intangible benefits, “soft benefits”, cannot. Good decision making requires factoring in both tangible and intangible costs and benefits. For example, laying off staff has a tangible benefit in cutting expenses. But if it leaves the rest of the workers worried about jobs and if these workers start looking for opportunities outside, the intangible costs may be quite significant.
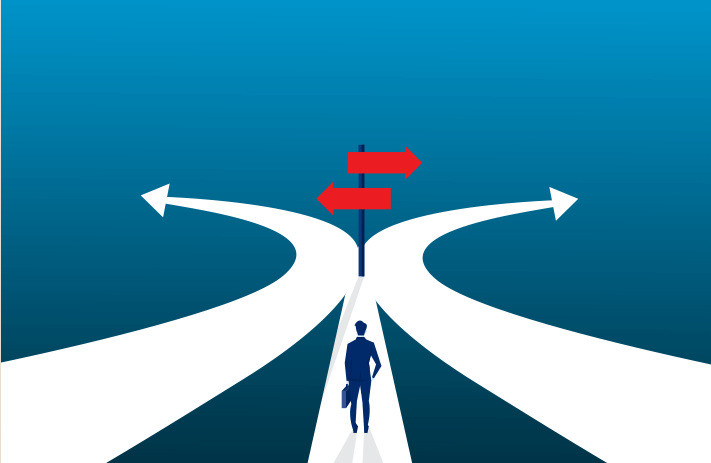
While many organizations use advanced analytics and AI regularly, few incorporate these tools into a holistic, systematic framework for decision making.
Chris Andrassy
Phase 3: Analyze
The analyze phase involves selecting the best alternative using payoff data and the probabilities associated with the outcomes. Nobel laureate Daniel Kahneman found that the best decisions are evaluated using numerical scores for competing alternatives and a yes-no decision on whether to choose a specific path. Though there are many analysis models to select the best alternative, four important ones based on risk and uncertainty are:
1. Expected Value (Realist)
Here you compute the expected value under each alternative based on the probability of the outcome and select the alternative with the largest expected value.
2. Maximax (Optimist)
This model selects the largest of the maximum payoffs among the given alternatives.
3. Maximin (Pessimist)
This model seeks the largest of the minimum payoffs among the competing alternatives. This model will provide the alternative which is the best of the worst payoffs.
4. Minimax-Regret (Opportunist)
Minimax-Regret decision making is based on looking back at the consequences of the decision. The minimax-regret criterion seeks the smallest of the maximum regrets among the alternatives where regret is the difference between the best payoff and the received payoff.
Phase 4: Realize
Once the optimal or best alternative is selected, the decision needs to be realized or implemented. However, this phase is often confronted with the threat of decision paralysis which can be addressed by quantifying the impact of the decision based on expected value and addressing pertinent biases such as loss aversion, group think, and confirmation bias.
Conclusion
We make decisions every moment of every day, both big and small, and almost every decision entails predicting the future. Research tells us that an average adult makes about 35,000 decisions every day. Even though every decision comes with some amount of risk and uncertainty, individuals and businesses who make rational decisions, unsurprisingly, tend to be more successful in their pursuits.
The DEAR model offers a structured, systematic approach to modeling decision making and mitigating the ever-present effects of cognitive bias.
About the Authors
Dr. Prashanth Southekal is managing principal of DBP Institute (www.dbp-institute.com), a data and analytics consulting, research, and education firm. He is also an advisor at Astral Insights. He is a Consultant, Author, and Professor. He has consulted for over 80 organizations including P&G, GE, Shell, Apple, and SAP. Dr. Southekal is the author of two books — “Data for Business Performance” and “Analytics Best Practices” — and writes regularly on data, analytics, and machine learning in Forbes.com, FP&A Trends, and CFO.University. His second book, ANALYTICS BEST PRACTICES was ranked the #1 analytics book of all time in May 2022 by BookAuthority. Apart from his consulting pursuits, he has trained over 3,000 professionals worldwide in Data and Analytics. Dr. Southekal is also an Adjunct Professor of Data and Analytics at IE Business School (Madrid, Spain). CDO Magazine included him in the top 75 global academic data leaders of 2022. He holds a PhD. from ESC Lille (FR) and an MBA from Kellogg School of Management (U.S.). He lives in Calgary, Canada with his wife, two children, and a high-energy Goldendoodle dog. Outside work, he loves juggling and cricket.
Christopher Andrassy is an entrepreneur and managing partner at Astral Insights, focused on transforming data into sustainable business value on a global scale. He began his career at PwC in New York City, supporting the digital transformation of mature organizations struggling to innovate in a hyper-competitive world. After experiencing the shortcomings of traditional analytics practices, he decided to begin a new chapter alongside colleagues and industry veterans. His departure from New York marked the inception of Astral Insights, a Raleigh-based decision intelligence firm helping mid-market and enterprise clients transform data into profit. Chris is also an investor focused on innovative technologies including synthetic biology, sustainable energy, and artificial intelligence. Outside of work, he is an avid musician, skier, traveler, and fitness enthusiast.